On Experiments for Causal Inference and System Identification
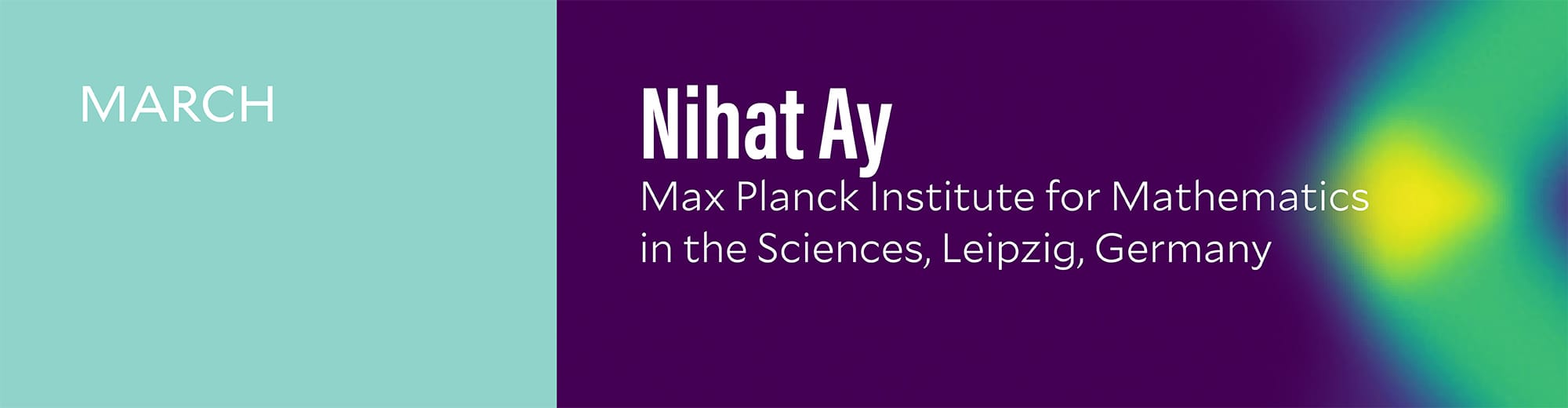
In the first part of his presentation, Professor Ay will provide an introduction to the field of causal networks. He will focus on instructive simple examples in order to highlight the core conceptual and philosophical ideas that underly the theory. This approach provides a clear semantics that allows us to distinguish between causation and correlation. It also allows us to study how cause-effect relations give rise to particular kinds of correlations, which can then be exploited for inferring causal relations from correlations. However, this kind of inference, which is based on observations only, turns out to be rather limited. In addition to observing a system, one can also apply experimental interventions and thereby reveal causal structures that would not follow from correlational information. This is one instance of system identification through experimental perturbations. Professor Ay will conclude my presentation with an extension of the interventional calculus by a “knockout calculus,” which is based on a kind of structural intervention. This allows us to reveal a more fine-grained causal description of the underlying system.
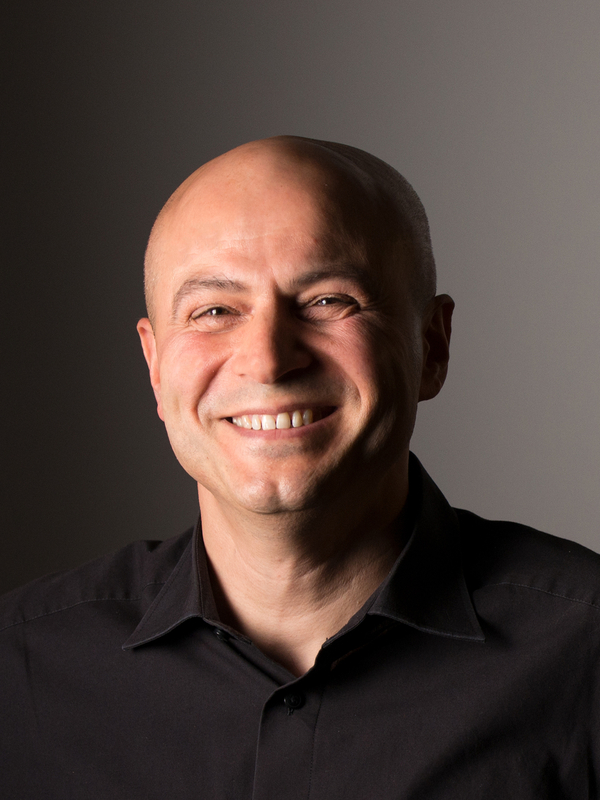
Nihat Ay
Nihat Ay studied mathematics and physics at the Ruhr University Bochum and received his Ph.D. in mathematics from the University of Leipzig in 2001. In 2003 and 2004, he was a postdoctoral fellow at the Santa Fe Institute and at the Redwood Neuroscience Institute (now the Redwood Center for Theoretical Neuroscience at UC Berkeley). After his postdoctoral stay in the USA, he became an assistant professor (wissenschaftlicher Assistent) at the Mathematical Institute of the Friedrich Alexander University in Erlangen. Since September 2005, he works as Max Planck Research Group Leader at the Max Planck Institute for Mathematics in the Sciences in Leipzig where he is heading the group Information Theory of Cognitive Systems. As professor of the Santa Fe Institute he is involved in research on complexity and robustness theory. Since December 2013, he has been affiliated with the Leipzig University as Honorary Professor for Information Geometry. He recently accepted an offer from the Hamburg University of Technology, where he is going to build up a new institute, the institute of Data Science Foundations, as part of the Technology and Innovation Center at the Hamburg Innovation Port (https://www.hamburg-innovation-port.com/).
The conversant for the post-talk conversation is Professor Kyle Cranmer, a Professor of Physics and Data Science at New York University. He is an experimental particle physicist working, primarily, on the Large Hadron Collider, based in Geneva, Switzerland. Professor Cranmer obtained his Ph.D. in Physics from the University of Wisconsin-Madison in 2005 and his B.A. in Mathematics and Physics from Rice University. He was awarded the Presidential Early Career Award for Science and Engineering in 2007 and the National Science Foundation’s Career Award in 2009. Professor Cranmer developed a framework that enables collaborative statistical modeling, which was used extensively for the discovery of the Higgs boson in July, 2012. His current interests are at the intersection of physics, statistics, and machine learning.