Causality at the Intersection of Simulation, Inference, Science, and Learning
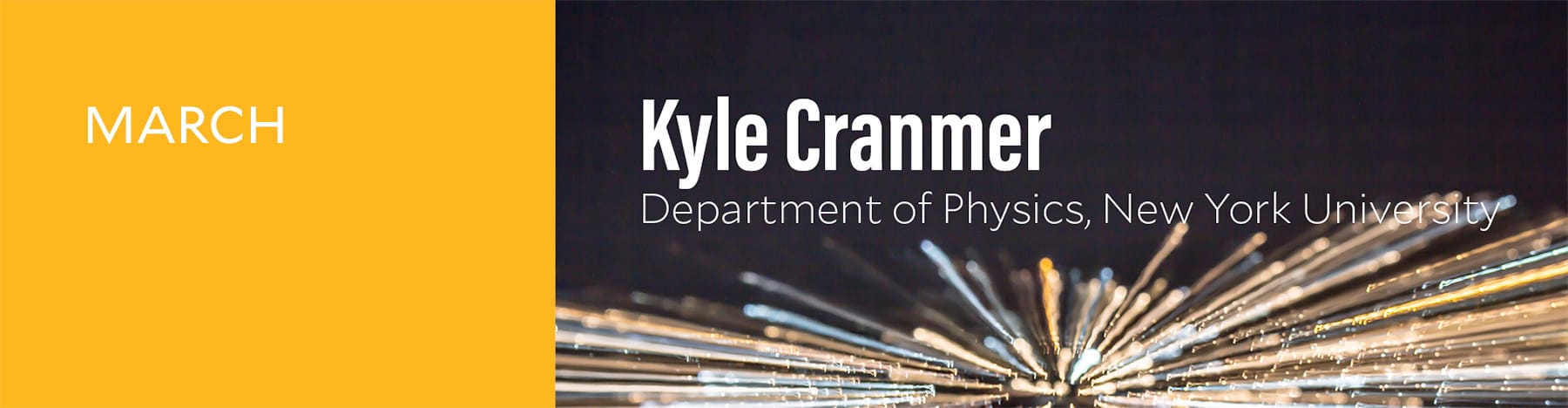
The sciences are replete with high-fidelity simulators: computational manifestations of causal, mechanistic models. Ironically, while these simulators provide our highest-fidelity physical models, they are not well suited for inferring properties of the model from data. In this talk, Professor Kyle Cranmer of the Department of Physics of New York University will describe the emerging area of simulation-based inference and describe how machine learning is being brought to bear on these challenging problems. It is then tempting to imagine the path towards developing an autonomous AI scientist also responsible for designing experiments and hypothesis generation. Interestingly, this framing brings a new perspective on the role of causality and agency that incorporates recent advances in machine learning and artificial intelligence.
The moderated post-talk conversation will take place on Wednesday, March 16 at 4 p.m., and the conversant will Zenna Tavares, Associate Research Scientist and Alan Kanzer Innovation Scholar at the Zuckerman Institute and Data Science Institute of Columbia University. Dr. Tavares is the first joint hire between two university-level institutes at Columbia University. His research aims to understand how humans reason, that is, how they come to derive knowledge from observing and interacting with the world. He also constructs computational and statistical tools that help advance his work on causal reasoning, probabilistic programming and other areas. Prior to Columbia University he was at MIT, where he received a Ph.D. in Cognitive Science and Statistics and was a Postdoctoral Research researcher in the Computer Science Artificial Intelligence Lab (CSAIL). Dr. Tavares’ work has received significant recognition including an International Fulbright Science and Technology Award for Outstanding Foreign Students.
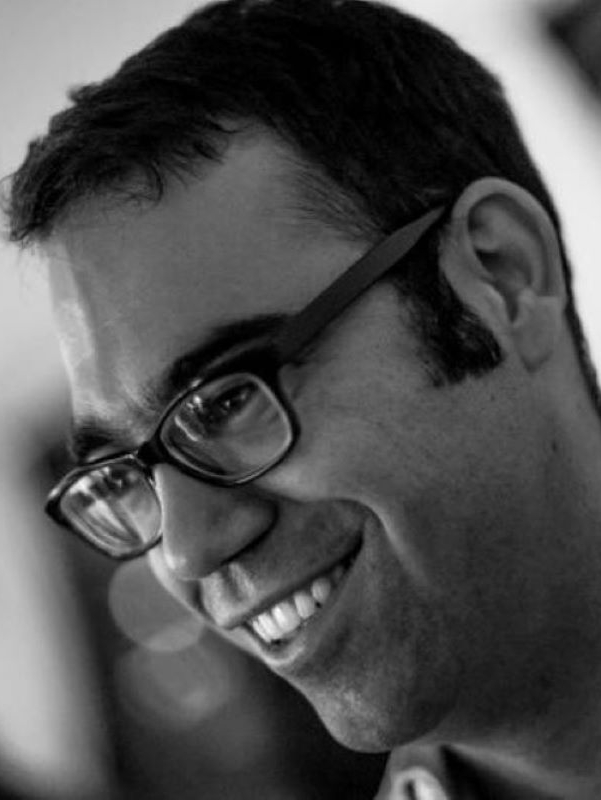
Kyle Cranmer
Professor Kyle Cranmer is a professor of physics at New York University; he is interested in particle physics, machine learning, statistics, open science, cyberinfrastructure, and science communication to the broader public. He graduated in 1999 from Rice University with his B.A. degree in physics and mathematics, earned his M.A. in 2002 from the University of Wisconsin-Madison, followed by his Ph.D. in 2005 from the same.