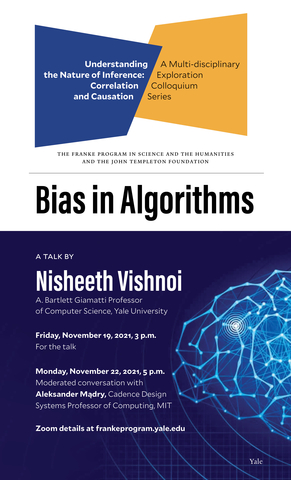
A key objective of Artificial Intelligence (AI) techniques is to learn from data how patterns can be associated with prediction power. To be able to extract inference from data as a tool to be able to implicate, authorize, and supervise various aspects of society is groundbreaking. In addition, for the hiring and public services sectors, identities, demographic attributes, preferences, and predicting future behavior as related to criminal justice and lending are all possible applications for these algorithms.
Recently, artificial algorithms have made significant progress toward replicating natural intelligence based on human-generated datasets that allow for the training of a machine learning model which is a blend of a computational and statistical approach. While these algorithms have led to significant economic and social growth, they have also been found to be biased. Some algorithms may reproduce and even amplify human biases, especially those affecting marginalized societies.
The objective world of artificial algorithms is shaped by social and human biases, and our challenge lies in developing algorithms without these biases and limitations. A bias can arise due to historical human biases or incomplete and unrepresentative data because if the training set is more representative of certain groups of people than others, then the predictions of the model may also be statistically worse for marginalized people. For a fair understanding of bias, a straightforward assessment of the set of outputs that the algorithm produces to check for abnormal results is insufficient. Ultimately, these fairness and accuracy considerations should be driven by discussions regarding ethical frameworks and reasonable guidelines for machine learning algorithms.
AI algorithms are fundamental to a healthy economy as they offer far greater clarity and transparency about the ingredients and motivations behind economic decisions, and therefore offer significantly better opportunities for growth. If, however, there is no mechanism that integrates technical diligence, justice, and transparency from design to execution, it may lead to an amplified unethical practice and discrimination.
In the talk, Professor Nisheeth Vishnoi will discuss how policies and ethics related to artificial intelligence can be altered to overcome these limitations and biases in human decision-making algorithms.
–Zahra Kanji