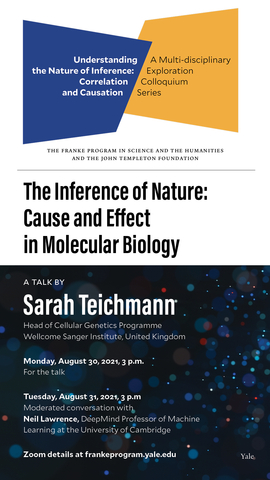
The study of computational biology, theoretical biology, and bioinformatics enable us to derive molecular and cellular components and their interactions. On this level of theory, computational biology aims to predict causal connections through correlations. This process of expressing the correlations of genes in the genome through millions of cells simultaneously results in very large data sets. In other words, differential equation-based systems in mechanistic models cannot handle this much data, thus we must use statistical, machine learning, deep learning, and artificial intelligence methods for correlation. We are privileged in molecular and cellular biology to be able to probe the systems we are studying experimentally. As a result, modeling and inference have always played some role in biology, and genetics is an example of it.
A molecular structure that is three-dimensional, such as the double helix, can be approached either using a crystal graphic experimental approach that publishes the x-ray diffraction pattern of crystals of DNA or via computational analysis that results from the pattern. In other words, experimental measurements rely on computation. Therefore, inference takes two forms: the first is a computation based on experimental measurements, the second is blending experimental data, and experimental data is critical to building models.
Professor Sarah Teichmann focused on three topics during her talk; she began by describing how to predict protein complex assembly. The second involved predicting cell types based on single-cell genomics. The third one dealt with using human cell atlases to predict COVID-19 infection and cell communication. A study she carried out showed that protein assembly pathways follow an orderly process, exactly like protein folding. To determine the relationships between proteins and genes, she inferred relationships between thousands of protein structures using biological principles, evolutionary relationships, and evolutionary sequences. Researchers have developed a method to map individual cells to spatial genomic analyses, and they have inferred the key type of inference needed from these analyses is cell type enforcement.
As she explained in her closing remarks, the human cell atlas is a consortium of international researchers involved in creating a comprehensive reference map of human cells by merging genomics information with spatial data. Now we are able to research single-cell genomics with big data, much like we used to do with protein structures, like AlphaFold. In view of its large size, this data requires only a computational inference for interpretation. Even so, mathematical algorithms must adapt to the technological advances in biological science in order to be able to interpret these enormous datasets.
– Gaurav Lohkna